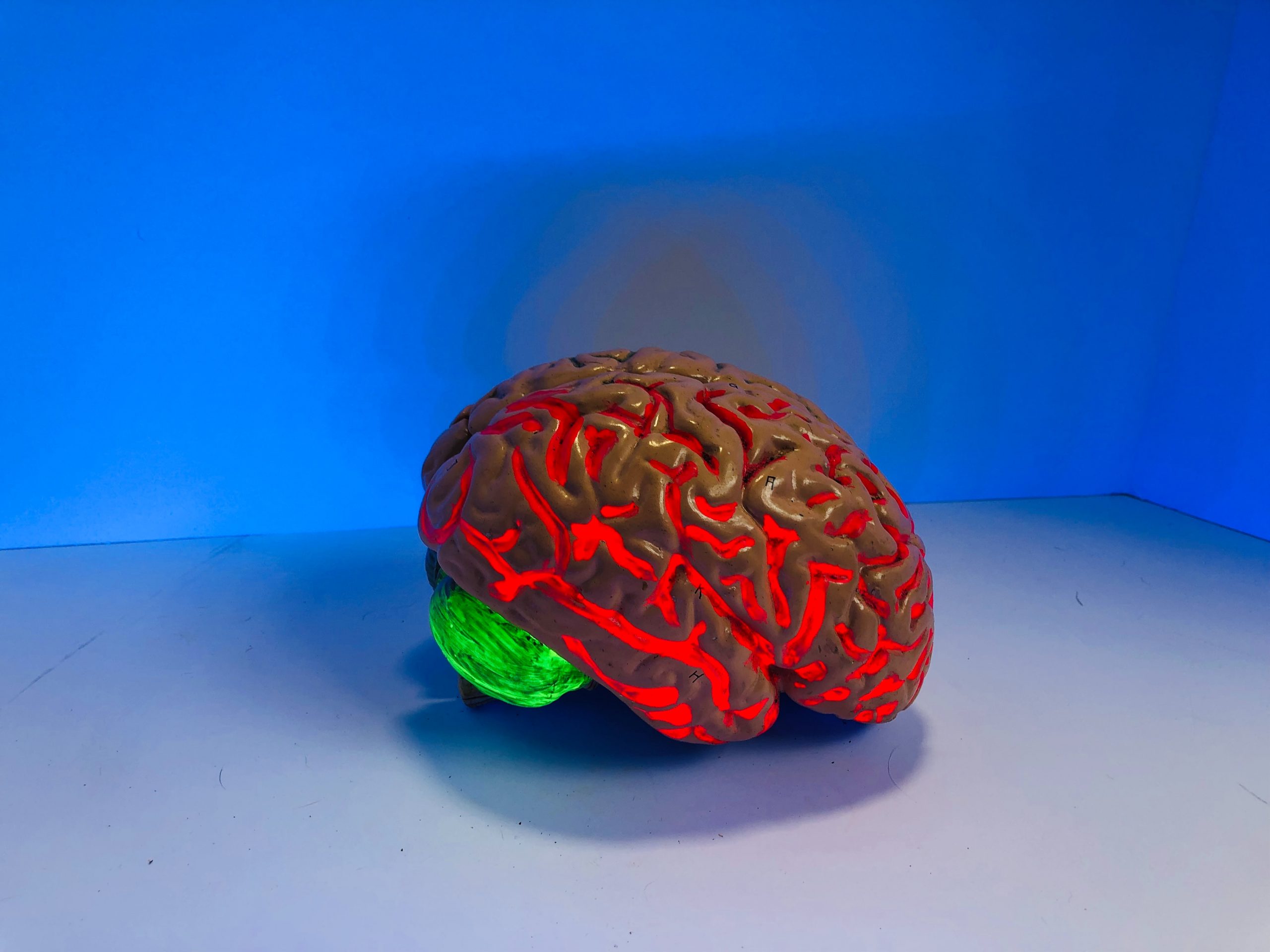
The National Institutes of Health grant will help researchers explore the use of machine learning for a minimally invasive system to detect Alzheimer's disease as early as possible. Credit: Natasha Connell/Unsplash.
Researchers to use $1.2 million grant to study early Alzheimer’s detection
Posted on October 27, 2022UNIVERSITY PARK, Pa — A team of Penn State-led researchers received a $1.2 million grant from the National Institutes of Health to help fund a project to develop a machine learning system for early Alzheimer’s disease detection. Alzheimer’s disease, a neurological condition and the most common form of dementia, affects nearly 6 million Americans, according to the Centers for Disease Control and Prevention.
According to the researchers, the system the system will provide a minimally invasive approach for detecting Alzheimer’s disease. Alzheimer’s disease (AD) is a severe neurodegenerative disorder characterized by progressive memory, cognitive impairment and personality changes, which can further evolve to dementia and death. Early detection allows doctors to give timely treatments and interventions for the patient.
As the nation’s population ages, the condition is becoming a growing health concern, according to Fenglong Ma, assistant professor of information sciences and technology and Institute for Computational and Data Sciences co-hire. Currently, doctors rely on several biomarkers — substances in an organism that can indicate the existence of a disease or condition — to detect Alzheimer’s disease. However, collecting data that inform about these biomarkers is expensive and can be time-consuming, added Ma, who is the lead principal investigator for the grant.
”Our team wants to contribute to a solution to these issues by developing a novel and minimally-invasive system,” said Ma. “By integrating a multimodal biosensing platform and a machine learning framework, we expect the system to improve early detection of Alzheimer’s disease and enhance AD detection accuracy.”
The team, which includes Penn State, MIT, Harvard University and Rice University researchers, plans to design a system that utilizes a variety of biosensors, including optical, mechanical and electrochemical nano-sensors, that can analyze biological samples — according to Sharon Huang, professor of information sciences and technology and Huck Institutes of the Life Sciences co-hire.
“Given different types of sensing data, for instance, data acquired from different biochemical markers in human body fluids, machine learning can perform feature selection and establish associations between an individual biomarker and Alzheimer’s disease, or between a set of biomarkers and the disease,” said Huang, who is a co-principal investigator for the grant.
“We hope our project can result in a minimally-invasive technique that can detect Alzheimer’s disease in its early stages,” Huang said. “The technique also has the potential to be high throughput, making it possible to be used in screening for the disease. We will also try our best to make the technique accurate, reducing false positives and false negatives in AD detection.”
Biosensing, machine learning partnership
According to Ma, biosensing data matches well with the capabilities of machine learning techniques and the combination of the two technologies could even pave the way to new discoveries for other conditions and diseases, he added.
“The biosensing platform will generate different types of sensing data, and machine learning aims to analyze these data to predict Alzheimer’s in the early stage,” Ma said. “Since the sensing data are so diverse — or heterogenous — advanced machine learning techniques can help model such data. Also, machine learning may help us identify some new AD biomarkers.”
Right now, the team is analyzing animal biological samples, but, if these initial inquiries prove successful, the team will move on to study human biological samples.
“After that, our next steps are to develop more inexpensive and non-invasive diagnosis methods for AD and incorporate more sensing data such as human vocal biomarkers and eye movement biomarkers,” said Ma.