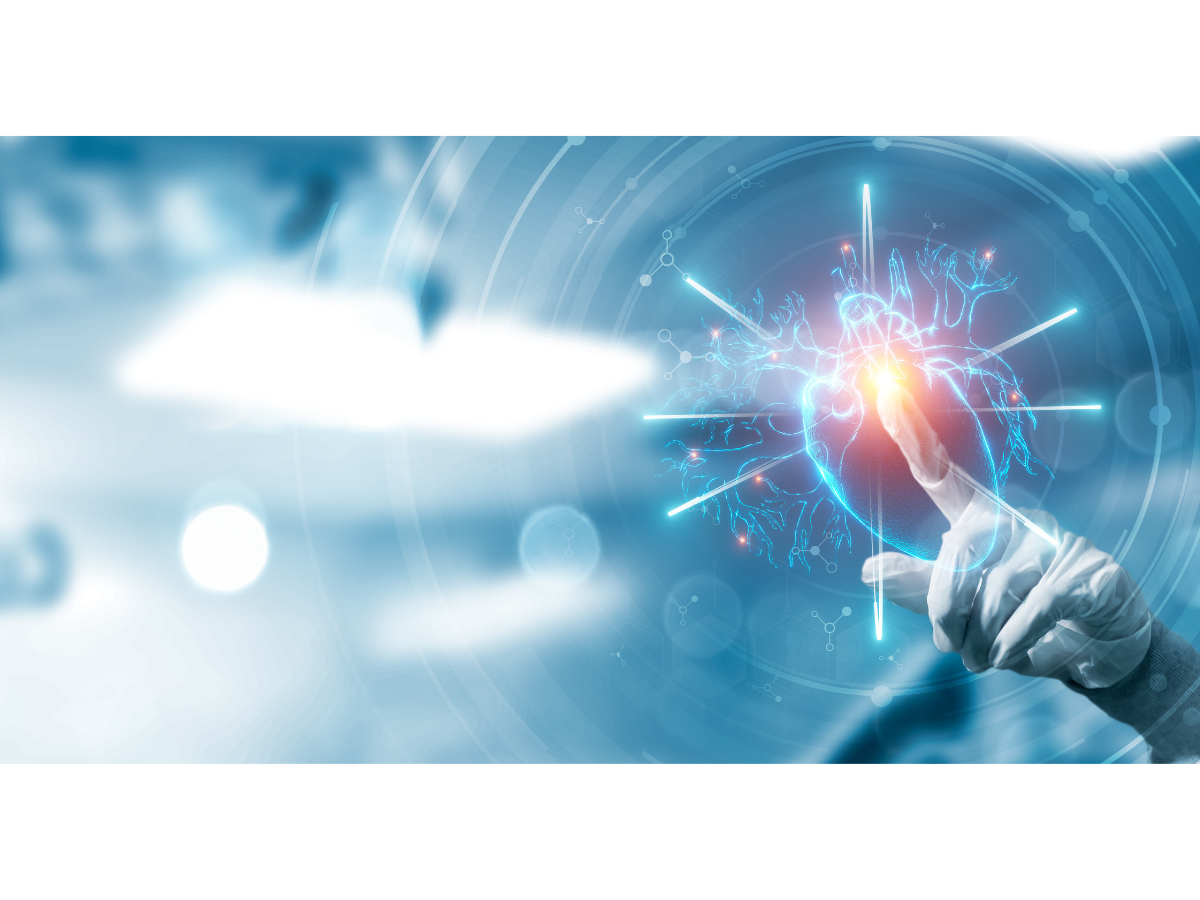
RISE engineers support cardiology collaboration
Posted on December 10, 2024Editor’s Note: A version of this article was originally published on Penn State News.
Penn State Institute for Computational and Data Sciences (ICDS) RISE engineers, with support from the Penn State Clinical and Translational Science Institute (CTSI)’s see grant program, were able to collaborate with University researchers and a team of physicians from the Penn State Heart and Vascular Institute on a project utilizing machine learning and existing electrocardiogram (ECG) data to help make more accurate predictions. The project, which has been ongoing since November, aims to develop novel algorithms for point-of-care, in-house use and for technology licensing.
Daniel Asante Otchere, ICDS research computing software engineer (RISE), under the supervision of Justin Petucci, ICDS R&D (RISE) engineer, is leading the artificial intelligence and computational support of the project alongside Ankit Maheshwari, assistant professor of medicine at Penn State and project lead researcher.
In September, an initial pilot study was published in the journal Heart Rhythm. The publication reported a model that could predict whether a patient with stroke of unknown cause would develop atrial fibrillation (AFib) by analyzing one heartbeat from a standard ECG test.
The research team wanted to see if they could use this standard test to predict AFib instead of relying on a loop recorder. The current standard of care is to implant a loop recorder, according to Penn State News. A loop recorder is a device that tracks heart activity to check for AFib and is placed under the skin. The team compiled a small data set using existing ECG data from Penn State comprising patients with cryptogenic stroke, or stroke without a clear cause, who had these loop recorders implanted. They also took data from 12-lead ECGs. Using machine learning algorithms, the team developed a model that could take the data and predict whether they would, or would not, develop AFib. The model correctly classified 80% of patients in the test cohort, Penn State News said.
Researchers are also aiming to expand the database, which would allow for broader applications according to Maheshwari. The multi-year project has the potential to have a broader impact by implementing predictive modeling in other heart-related areas, for example, predicting when to use pacemakers in patients undergoing transcatheter aortic valve replacement procedures.
“This could lead to better patient selection and outcomes, helping doctors identify who is most likely to benefit from the procedure and who might face complications,” Maheshwari said. “These advancements highlight the great potential of machine learning to make heart care more efficient.”
The team is also focused on validating their predictive models. The goal is to have a fully functional database enabling them to conduct larger-scale studies, as well as to potentially apply for additional funding to support randomized, controlled trials. The initial funding was awarded through CTSI’s seed grant program for 2024-2025, alongside various other projects using machine learning. The goal of CTSI’s program is to establish collaborations needed to realize the potential of AI in biomedical and health research. CTSI’s Informatics Core, the National Center for Advancing Translational Sciences and the National Institutes of Health, through grant UL1 TR002014, are also supporting this research.
“Our efforts not only hold promise for improving patient care but also represent a paradigm shift in how cardiovascular diseases may be diagnosed and managed in the coming years,” Maheshwari said.
Share
Related Posts
- Professor receives NSF grant to model cell disorder in heart
- Featured Researcher: Nick Tusay
- Multi-institutional team to use AI to evaluate social, behavioral science claims
- NSF invests in cyberinfrastructure institute to harness cosmic data
- Center for Immersive Experiences set to debut, serving researchers and students
- Distant Suns, Distant Worlds
- CyberScience Seminar: Researcher to discuss how AI can help people avoid adverse drug interactions
- AI could offer warnings about serious side effects of drug-drug interactions
- Taking RTKI drugs during radiotherapy may not aid survival, worsens side effects
- Cost-effective cloud research computing options now available for researchers
- Costs of natural disasters are increasing at the high end
- Model helps choose wind farm locations, predicts output
- Virus may jump species through ‘rock-and-roll’ motion with receptors
- Researchers seek to revolutionize catalyst design with machine learning
- Resilient Resumes team places third in Nittany AI Challenge
- ‘AI in Action’: Machine learning may help scientists explore deep sleep
- Clickbait Secrets Exposed! Humans and AI team up to improve clickbait detection
- Focusing computational power for more accurate, efficient weather forecasts
- How many Earth-like planets are around sun-like stars?
- SMH! Brains trained on e-devices may struggle to understand scientific info
- Whole genome sequencing may help officials get a handle on disease outbreaks
- New tool could reduce security analysts’ workloads by automating data triage
- Careful analysis of volcano’s plumbing system may give tips on pending eruptions
- Reducing farm greenhouse gas emissions may plant the seed for a cooler planet
- Using artificial intelligence to detect discrimination
- Four ways scholars say we can cut the chances of nasty satellite data surprises
- Game theory shows why stigmatization may not make sense in modern society
- Older adults can serve communities as engines of everyday innovation
- Pig-Pen effect: Mixing skin oil and ozone can produce a personal pollution cloud
- Researchers find genes that could help create more resilient chickens
- Despite dire predictions, levels of social support remain steady in the U.S.
- For many, friends and family, not doctors, serve as a gateway to opioid misuse
- New algorithm may help people store more pictures, share videos faster
- Head named for Ken and Mary Alice Lindquist Department of Nuclear Engineering
- Scientific evidence boosts action for activists, decreases action for scientists
- People explore options, then selectively represent good options to make difficult decisions
- Map reveals that lynching extended far beyond the deep South
- Gravitational forces in protoplanetary disks push super-Earths close to stars
- Supercomputer cluster donation helps turn high school class into climate science research lab
- Believing machines can out-do people may fuel acceptance of self-driving cars
- People more likely to trust machines than humans with their private info
- IBM donates system to Penn State to advance AI research
- ICS Seed Grants to power projects that use AI, machine learning for common good
- Penn State Berks team advances to MVP Phase of Nittany AI Challenge
- Creepy computers or people partners? Working to make AI that enhances humanity
- Sky is clearing for using AI to probe weather variability
- ‘AI will see you now’: Panel to discuss the AI revolution in health and medicine
- Privacy law scholars must address potential for nasty satellite data surprises
- Researchers take aim at hackers trying to attack high-value AI models
- Girls, economically disadvantaged less likely to get parental urging to study computers
- Seed grants awarded to projects using Twitter data
- Researchers find features that shape mechanical force during protein synthesis
- A peek at living room decor suggests how decorations vary around the world
- Interactive websites may cause antismoking messages to backfire
- Changing how government assesses risk may ease fallout from extreme financial events
- Penn State’s Leadership in AI Research
- ICS co-sponsors Health, Environment Seed Grant Program
- Symposium at U.S. Capitol seeks solutions to election security